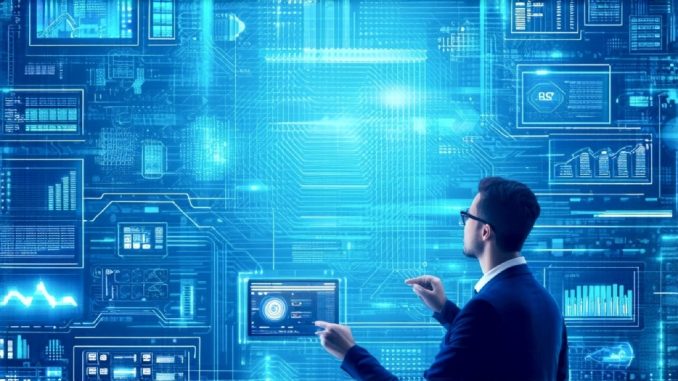
Join our daily and weekly newsletters for the latest updates and exclusive content on industry-leading AI coverage. Learn More
Hallucination is a risk that limits the real-world deployment of enterprise AI.
Many organizations have attempted to solve the challenge of hallucination reduction with various approaches, each with varying degrees of success. Among the many vendors that have been working for the last several years to reduce the risk is Vectara. The company got its start as an early pioneer in grounded retrieval, which is better known today by the acronym Retrieval Augmented Generation (RAG). An early promise of RAG was that it could help reduce hallucinations by sourcing information from provided content.
While RAG is helpful as a hallucination reduction approach, hallucinations still occur even with RAG. Among existing industry solutions most solutions focus on detecting hallucinations or implementing preventative guardrails, Vectara has unveiled a fundamentally different approach: automatically identifying, explaining and correcting AI hallucinations through what it calls guardian agents inside of a new service called the Vectara Hallucination Corrector.
The guardian agents are functionally software components that monitor and take protective actions within AI workflows. Instead of just applying rules inside of an LLM, the promise of guardian agents is to apply corrective measures in an agentic AI approach that improves workflows. Vectara’s approach makes surgical corrections while preserving the overall content and providing detailed explanations of what was changed and why.
The approach appears to deliver meaningful results. According to Vectara, the system can reduce hallucination rates for smaller language models under 7 billion parameters, to less than 1%.
“As enterprises are implementing more agentic workflows, we all know that hallucinations are still an issue with LLMs and how that is going to exponentially amplify the negative impact of making mistakes in an agentic workflow is kind of scary for enterprises,” Eva Nahari, chief product officer at Vectara told VentureBeat in an exclusive interview. “So what we have set out as a continuation of our mission to build out trusted AI and enable the full potential of gen AI for enterprise… is this new track of releasing guardian agents.”
The enterprise AI hallucination detection landscape
Every enterprise wants to have accurate AI, that’s not a surprise. It’s also no surprise that there are many different options for reducing hallucinations.
RAG approaches help to reduce hallucinations by providing grounded responses from content but can still yield inaccurate results. One of the more interesting implementations of RAG is one from the Mayo Clinic which uses a ‘reverse RAG‘ approach to limit hallucinations.
Improving data quality as well as how vector data embeddings are created is another approach to improving accuracy. Among the many vendors working on that approach is database vendor MongoDB which recently acquired advanced embedding and retrieval model vendor Voyage AI.
Guardrails, which are available from many vendors including Nvidia and AWS among others, help to detect risky outputs and can help with accuracy in some cases. IBM actually has a set of its Granite open-source models known as Granite Guardian that directly integrate guardrails as a series of fine-tuning instructions, to reduce risky outputs.
Using reasoning to validate output is another potential solution. AWS claims that its Bedrock Automated Reasoning approach catches 100% of hallucinations, though that claim is difficult to validate.
Startup Oumi offers another approach, validating claims made by AI on a sentence by sentence basis by validating source materials with an open-source technology called HallOumi.
How the guardian agent approach is different
While there is merit to all the other approaches to hallucination reduction, Vectara claims its approach is different.
Rather than just identifying if a hallucination is present and then either flagging or rejecting the content, the guardian agent approach actually corrects the issue. Nahari emphasized that the guardian agent takes action.
“It’s not just a learning on something,” she said. “It’s taking an action on behalf of someone, and that makes it an agent.”
The technical mechanics of guardian agents
The guardian agent is a multi-stage pipeline rather than a single model.
Suleman Kazi, machine learning tech lead at Vectara told VentureBeat that the system comprises three key components: a generative model, a hallucination detection model and a hallucination correction model. This agentic workflow allows for dynamic guardrailing of AI applications, addressing a critical concern for enterprises hesitant to fully embrace generative AI technologies.
Rather than wholesale elimination of potentially problematic outputs, the system can make minimal, precise adjustments to specific terms or phrases. Here’s how it works:
A primary LLM generates a response
Vectara’s hallucination detection model (Hughes Hallucination Evaluation Model) identifies potential hallucinations
If hallucinations are detected above a certain threshold, the correction agent activates
The correction agent makes minimal, precise changes to fix inaccuracies while preserving the rest of the content
The system provides detailed explanations of what was hallucinated and why
Why nuance matters for hallucination detection
The nuanced correction capabilities are critically important. Understanding the context of the query and source materials can make the difference between an answer being accurate or being a hallucination.
When discussing the nuances of hallucination correction, Kazi provided a specific example to illustrate why blanket hallucination correction isn’t always appropriate. He described a scenario where an AI is processing a science fiction book that describes the sky as red, instead of the typical blue. In this context, a rigid hallucination correction system might automatically “correct” the red sky to blue, which would be incorrect for the creative context of a science fiction narrative.
The example was used to demonstrate that hallucination correction needs contextual understanding. Not every deviation from expected information is a true hallucination – some are intentional creative choices or domain-specific descriptions. This highlights the complexity of developing an AI system that can distinguish between genuine errors and purposeful variations in language and description.
Alongside its guardian agent, Vectara is releasing HCMBench, an open-source evaluation toolkit for hallucination correction models.
This benchmark provides standardized ways to evaluate how well different approaches correct hallucinations. The goal of the benchmark is to help the community at large, as well as to help enable enterprises to evaluate hallucination correction claims accuracy, including those from Vectara. The toolkit supports multiple metrics including HHEM, Minicheck, AXCEL and FACTSJudge, providing comprehensive evaluation of hallucination correction effectiveness.
“If the community at large wants to develop their own correction models, they can use that benchmark as an evaluation data set to improve their models,” Kazi said.
What this means for enterprises
For enterprises navigating the risks of AI hallucinations, Vectara’s approach represents a significant shift in strategy.
Instead of just implementing detection systems or abandoning AI in high-risk use cases, companies can now consider a middle path: implementing correction capabilities. The guardian agent approach also aligns with the trend toward more complex, multi-step AI workflows.
Enterprises looking to implement these approaches should consider:
Evaluating where hallucination risks are most critical in their AI implementations.
Considering guardian agents for high-value, high-risk workflows where accuracy is paramount.
Maintaining human oversight capabilities alongside automated correction.
Leveraging benchmarks like HCMBench to evaluate hallucination correction capabilities.
With hallucination correction technologies maturing, enterprises may soon be able to deploy AI in previously restricted use cases while maintaining the accuracy standards required for critical business operations.
Be the first to comment